A few months ago, Netflix estimated that its recommendation engine is worth nearly $1 billion!
This is because Netflix’s terrific AI-driven recommendation engine accounts for more than 80% of the TV shows discovered on the platform. Netflix uses its recommendation system to bank on customer retention, its biggest ROI being the regular flow of a recurring subscription model.
‘‘If we create a more compelling service by offering better personalized recommendations, we induce members who were on the fence to stay longer, and improve retention.’’
Nowadays, integrating a smart AI-driven recommender into your audio/video streaming platform has become a must-have feature. All big brands have started using high-end recommenders to drive more traffic and increase revenue.
Role of Big Data
Recommender systems and ‘big data’ have a deep connection. Big data literally translates into an extremely large chunk of information or data that is processed by optimized algorithms for shaping better business strategies and outcomes. In a nutshell, even the most advanced recommenders cannot be effective without big data.
Top-notch OTT brands collect millions of data points from their users and use machine learning to analyze and refine the algorithms to rank content according to your preferences. Machine learning helps automate millions of decisions based on user activities. It takes several factors into consideration while predicting videos from its catalog such as:
- Interactions such as your browsing history or your ratings of other titles
- Other users’ interactions with similar tastes
- Relevant information like genre, categories, titles, the cast of titles
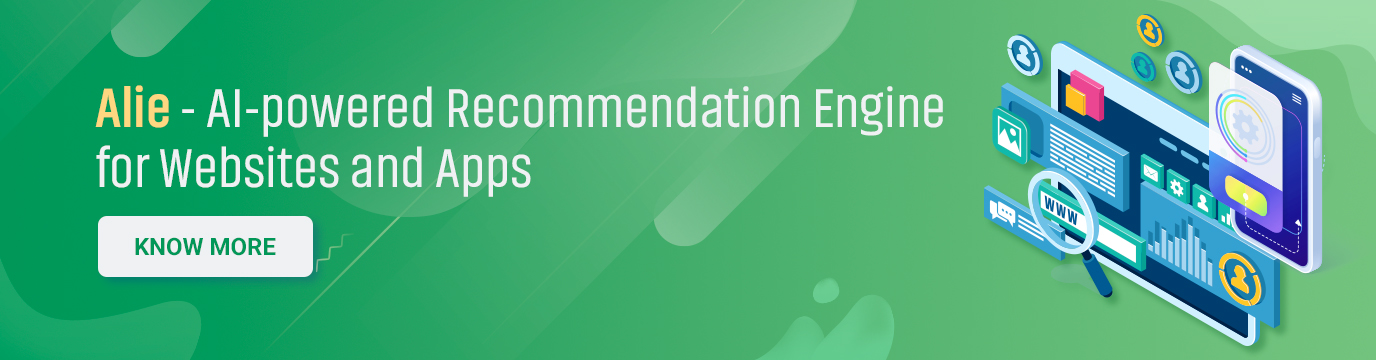
How does a recommendation system work?
A recommendation system understands human behavior and suggests content on that basis. A smart recommendation system like Alie uses sophisticated machine learning algorithms to offer your consumers with the most accurate suggestions.
- Collection of data – The first step of a recommender system starts with collecting data. It collects both implicit and explicit data from its users. Explicit data are those which are directly provided by the users such as ratings, comments, preferred genre, etc. On the other hand, implicit data is created by the recommender system itself with the browsing history, click-throughs, watch list events, etc, of each user.
- Storage of data– After collection of data, a recommender system stores data. The storage space should always be a well-managed one where any data can be stored securely.
Our recommender system, Alie uses a highly scalable cloud-based database, MongoDB, to make the data processing more simplified and secure.
- Analyzing data– After collecting explicit and implicit data and storing the same into the database, the recommender system starts analyzing the stored data.
Analyzing data is an important step towards accurate predictions as similar data is filtered out leading to more precise recommendations.
Alie uses an Artificial Intelligence-based advanced algorithm to process the stored data, filter out irrelevant ones and make recommendations based on users’ interests. Filtering data– It is the final step where the necessary data will be filtered from the entire database and only the relevant ones will be shown to your viewers.
A recommender system basically uses three different methods for filtering data and predicting accurate recommendations: Content-Based Filtering, Collaborative Filtering and Hybrid Filtering:
- Content-based Filtering: Content-based recommendation systems use their knowledge of each content (metadata of each content like title, genre, language, etc) to recommend new products.
For example, if you like watching movies starring Christian Bale, the recommender will start recommending movies like the Batman series, Ford vs Ferrari and all other movies starring Christian Bale.
- Collaborative Filtering: Collaborative Filtering is done based on historical data. The core assumption here is that the users who have agreed in the past tend to also agree in the future.
For example, Ron likes movies X, Y, and Z; and Rina likes movies W, X and Y. In this scenario, the collaborative filtering based algorithm will predict that Ron will also like the movie W and Rina will also like Z because of their similar liking towards X and Y.
- Hybrid Filtering: Hybrid filtering is a collaboration of both of the above-mentioned filtering processes. It recommends content to the viewers based on their own likings and the comparison between the habits of different viewers.
Now that you have got a fair idea about how a recommendation engine works, let us look at some of the advantages of deploying a smart recommender:
What are the top benefits of using a recommender system?
Here are the 4 benefits that streaming businesses can achieve using recommendation engines:
- Increase in revenue– Netflix has confessed to save about $1 billion every year due to its smart recommendation engine. An accurate recommendation system can recommend binge-worthy content for end-users so that they can enjoy hours of non-stop streaming, which in turn helps in subscriber retention, boosting your revenue.
- Personalization– A good recommender system helps massively towards personalization- because it curates a list of likeable content for the viewer considering their choice, watching patterns and browsing history. So, whenever they open your streaming app, their homepage gets filled with relevant content that they would like to watch.
- Customer satisfaction– Your customers will undoubtedly be more satisfied when they are able to find relevant content based on your platform that matches their tastes.
- Customer engagement– Consumers end up being more engaged in the website when personalized recommendations are made. This enhances customer engagement as they spend more time on your platform watching their favorite movies, TV shows, etc.
You too can integrate a powerful AI-powered recommendation system like Alie into your audio/video platform to personalize your viewers’ user experience.
Take a 14-Day Free Trial, now!
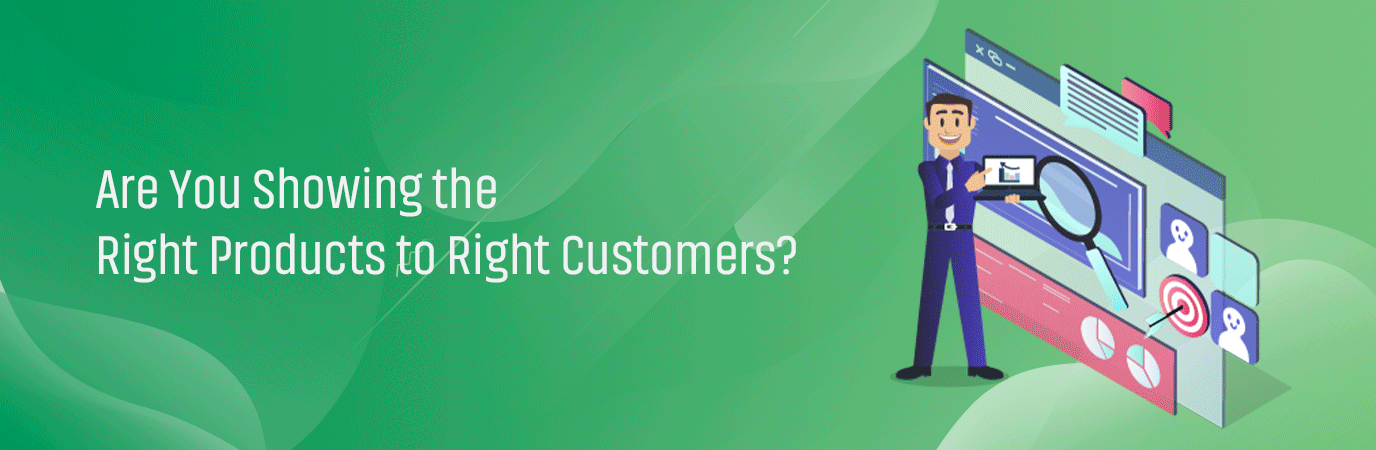
Add your comment