We all are aware of the fact that the Recommendation Engine has taken the world by storm. As customers, we are sometimes not even familiar with the recommendations/suggestions we get while using some website or mobile application. We cannot blame it because, more often than not, we are on a website, but we don’t know what we want to do there. Don’t believe it? Try to remember the last time you wanted to listen to songs but didn’t know which one to hear, so you opened the music application Spotify and decided which song to listen to based on the options available.
So, it is safe to say that the recommendation engine has become a part of our lives knowingly or unknowingly. However, have you ever noticed that there is one song on Spotify that you love, but not many people do; it never pops up in any of the lists made by Spotify. Why? Because recommendation engines thrive on feedback from users. If a product does not have a considerable amount of input from the users, the chances are that it might go unnoticed even if the product is extraordinary. This is where the quality products over quantity debates come into play.
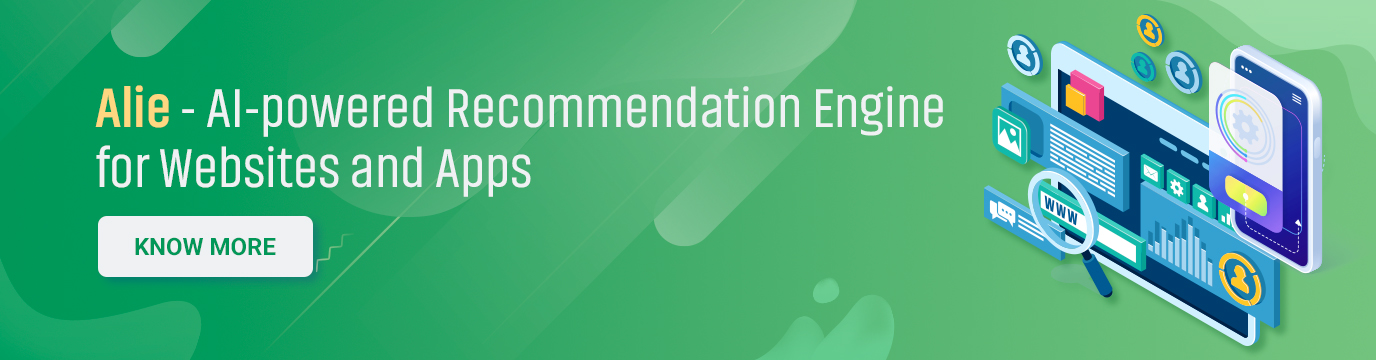
How does a Recommendation Engine Work?
To debate on anything related to recommendation engines, it is vital to understand how it works. There are mainly three steps that come under it –
Data Acquisition
As mentioned above, recommendation engines thrive on data. So, data acquisition is the first step in the process of recommending. It is a process of storing, filtering, and removing data that are not necessary. It can only be obtained when you allow your website to integrate with the recommendation engine or give your storage access.
Data Analysis
Once the data is acquired, the next step is to analyze it and shelf it in clusters. It is done through various methods involving machine learning. Some of the standard techniques are collaborative filtering and content-based filtering.
Also read : How does a Recommendation Engine use Predictive Analysis?
Recommendation
The last step is to identify the customer’s needs and then give recommendations to them. Any website owner who cares about his/her work must be curious to know which products are being recommended to which user. You can see all the activities of a recommendation engine like the image below if you use Alie.

Quality over Quantity Products in a Recommendation Engine
When a recommendation engine analyzes the data, it usually does so based on the inputs given by a user. What a user is browsing, their likes and dislikes, and what they purchase. When the recommendation engine follows item-based collaborative filtering, even then it has to deal with the products with some user feedback.
For products that are new or do not have any user activity makes it challenging for the recommendation engine to track it and then eventually suggest it to users. So, it is essential to have products/services that your target audience will enjoy.
Wrapping Up
However, there is one easy way to tackle this problem. It is to employ a recommendation engine where you can add your own algorithm. You can do this on Alie – a recommendation engine platform. Try its 14-day free trial to know more about it.
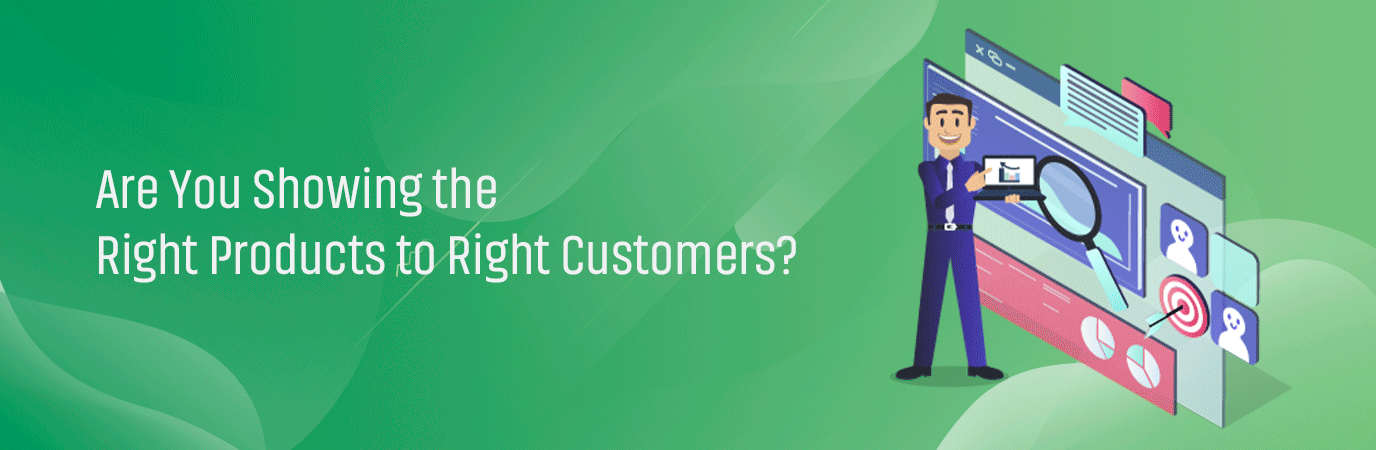
Add your comment