Ecommerce businesses nowadays offer a wide variety of products or items to their customers. Consumers on the other side are flooded with lots of choices to select from. The product recommendation engine has helped customers save their time going through all of the items sitewide and find the products they need quickly and easily. Due to time constraints, most of the users were not able to find relevant products and as a result, they end up leaving the website without any purchase. Offering personalized product recommendations have helped consumers enjoy a hassle-free shopping experience. These personalized recommendations are generated from users’ past browse and purchase behavior and product information.
Product recommendation engines are one of the most important tools for any eCommerce website or application. It increases conversions, boosts user engagement and average order value. It gives an assurance that your products are being excellently and continuously recommended to the end-users.
How has the Product Recommendation engine changed the face of marketing?
Consumers who come across high-quality product recommendations are 70% more probable to get converted because the product recommendation engine acts as a live salesperson.
In a traditional marketing environment, salespersons were the core of any business. Good Salespeople offered a high success rate to retail outlets. However, eCommerce is all about the self-guided experience. Customers navigate through the website and find out their favorite products with discounts and complete their purchase process on their own. Customers always prefer recommendations while shopping because it offers a personalized shopping experience and seems like someone is helping them who knows their tastes, likes, and dislikes.
Earlier, online shopping included a very little amount of personalization. All transactions were robotic and were the same for each consumer. Businesses nowadays understand that personalizing the user experience is vital to achieving success. And essentially integrating a recommendation engine can help businesses attain victory.
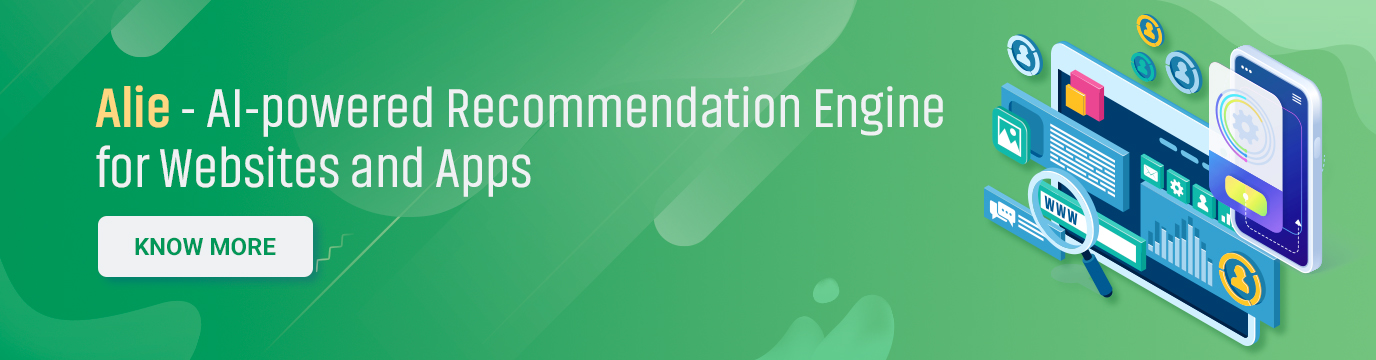
Product Recommendation Engine – An Overview
A product recommendation engine is a filtering system that predicts and shows the items that a user would like to buy. It uses algorithms and data to recommend the most relevant items to any particular user. It is an important tool for businesses to search, map, and provide users with relevant chunks of information based on their preferences and tastes.
For example – Amazon uses a recommender engine as a targeted marketing tool. Amazon’s recommender system suggests many products to the users based on their browsing behavior.
How does a product recommendation system work?
Machine learning algorithms are used in recommender systems to identify exactly what kind of products and services shoppers are interested in. The platform targets individual users using their search patterns and preferences to provide relevant products. It uses three types of filtering methods to categorize users and recommend their favorite items. These are:
- Content-based filtering
- Collaborative filtering
- Hybrid filtering
Content-based filtering
This method is based on the description of an item and a profile of the user’s preferred choice. It uses keywords to describe the items and the type of items any particular user likes.
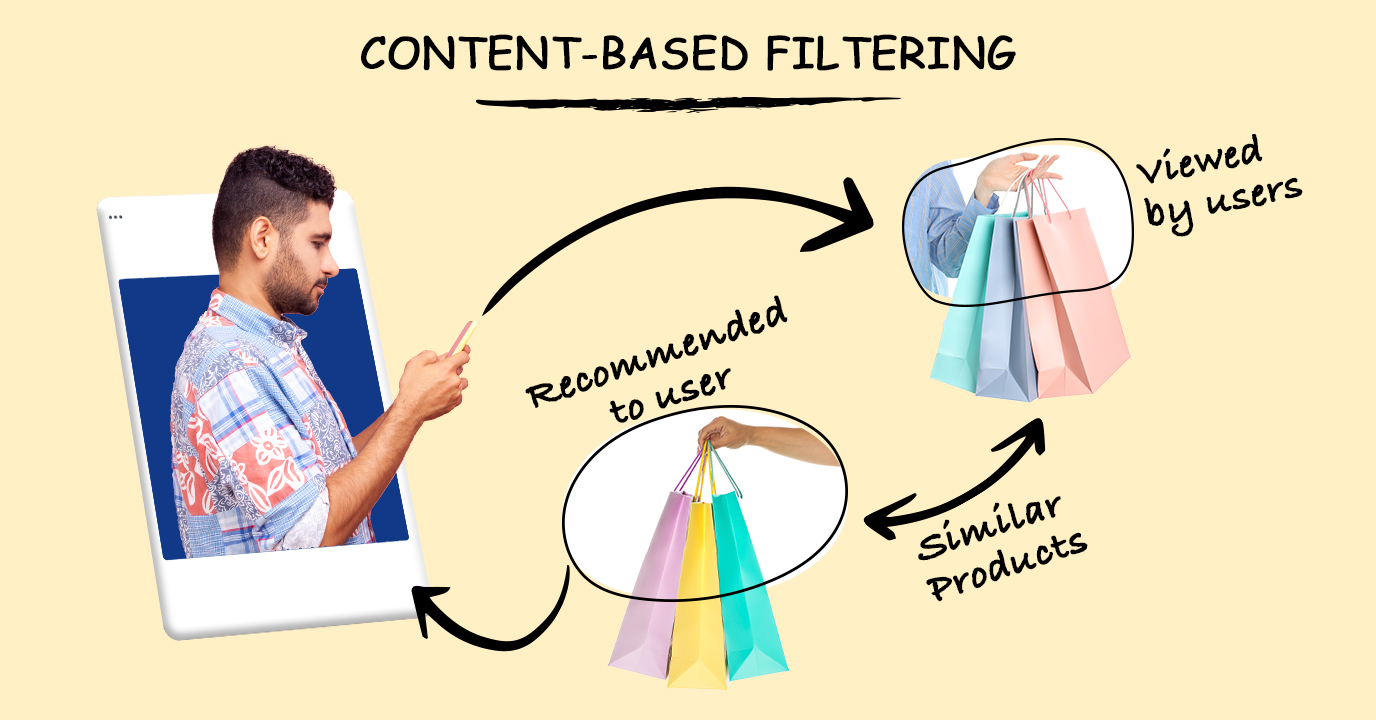
Collaborative filtering
This method usually collects and analyzes information on users’ behaviors, movements, or preferences, and predicts what they like based on their similarities with other users.
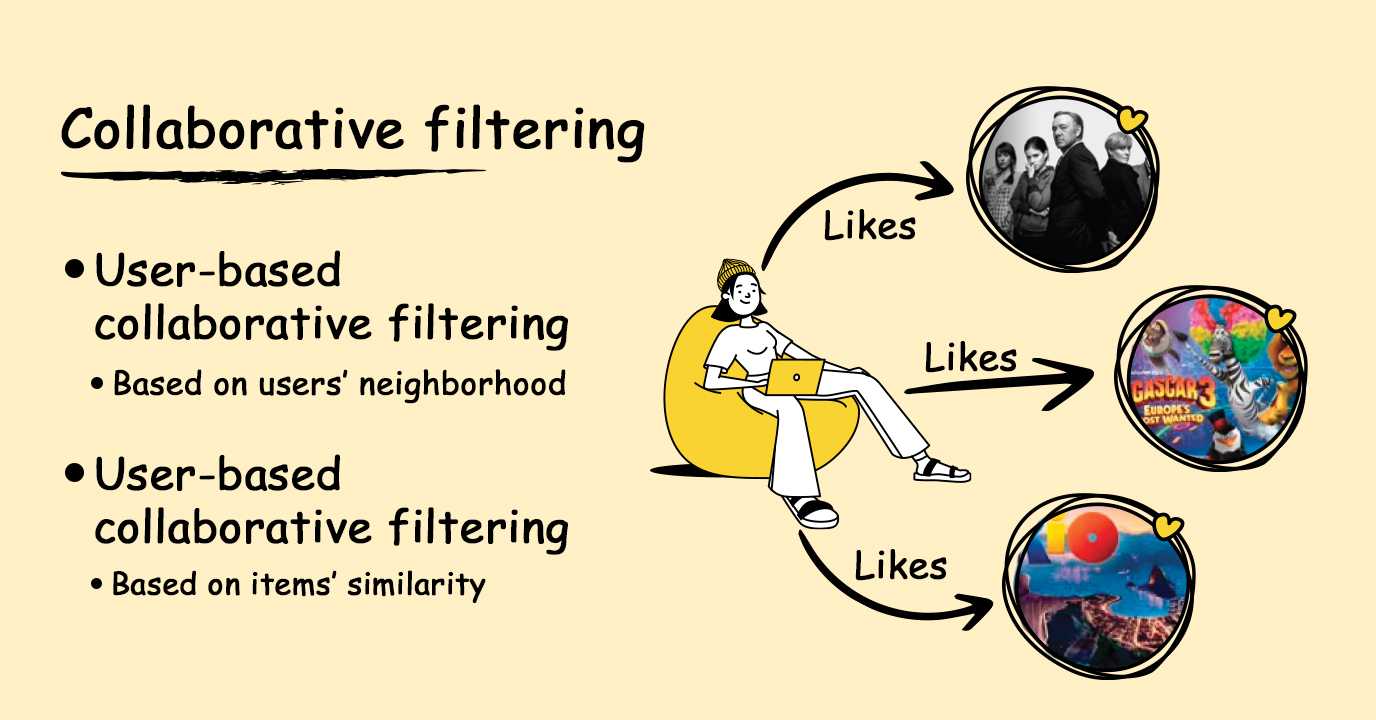
Hybrid Filtering
Hybrid Filtering is the mix of both filtering methods i.e., content-based and collaborative filtering. It recommends items to users by both using metadata and analyzing users’ behavior.
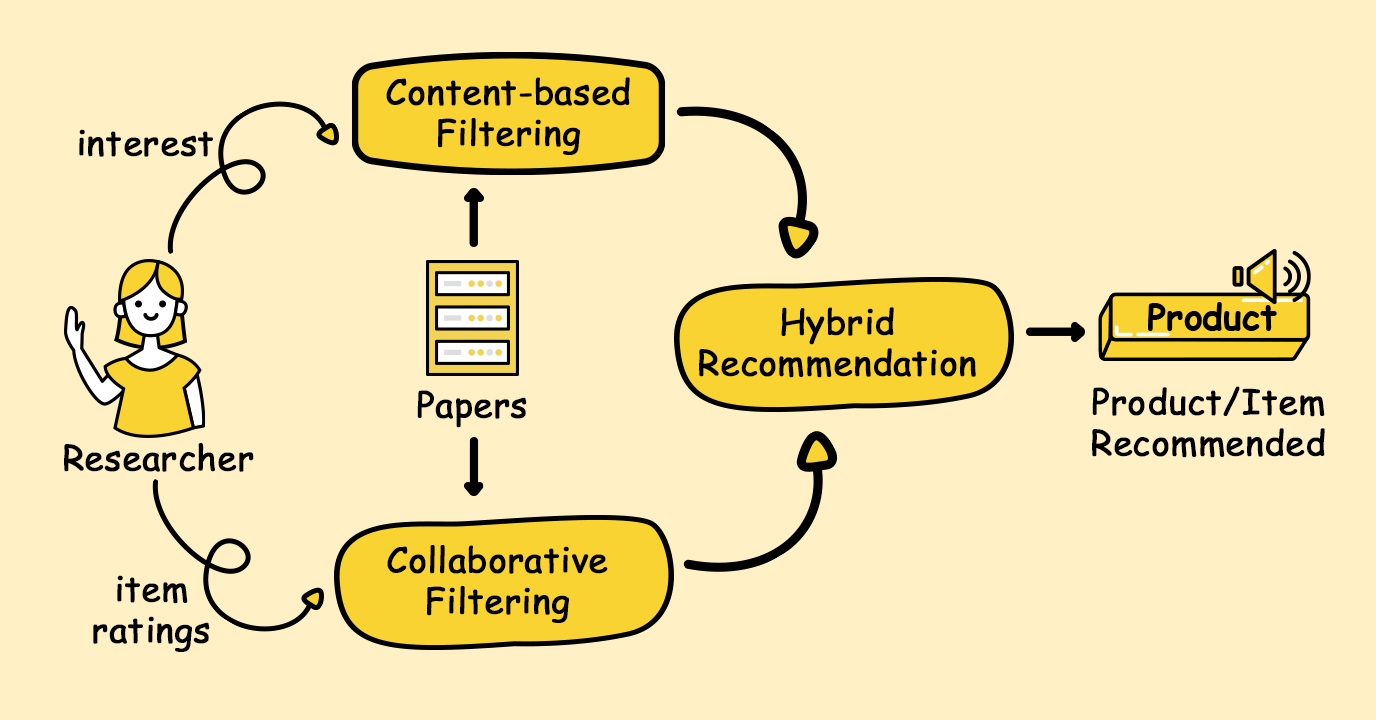
4 Phases That Every Product Recommendation Engine Goes Through
Collecting the data
Initially a recommender system collects the data for creating recommendations. Data has been classified into two categories i.e., explicit and implicit ones. Explicit data can be ratings and comments provided by users. Implicit data can be search logs, order and return history, clicks, page views, and cart events.
Storing the data
The recommender system then stores the data in the database. A scalable and managed database decreases the number of needed tasks to a minimum and focuses on the recommendation itself.
Analyzing the data
It is important for a recommender system to find items with similar user engagement data and hence analyzing the data with different methods becomes a crucial phase for any recommendation engine.
Filtering the data
The last but the most important phase is filtering the data to provide relevant recommendations. As mentioned above, the recommender system uses 3 types of filtering methods and recommends precisely to the audience.
Conclusion
There is no doubt that the product recommendation engine has gained immense popularity and plays a vital role in the novel digital era. In order to be successful in this cutthroat marketplace, eCommerce businesses need to integrate recommendation engines with their website and platforms. Thanks to AI, the recommender system has improved their efficiency and are efficiently helping businesses grow exponentially.
Help Shoppers Find their Favorite Product with Alie!
Alie is an AI-powered product recommendation engine that helps shoppers experience a personalized buying experience by providing them with real-time recommendations. Its unique machine learning algorithm is designed to analyze user data and suggest personalized product recommendations in real-time. Start a 14-day free trial to experience how Alie can seamlessly integrate with your website and recommend products flawlessly to your end-users.
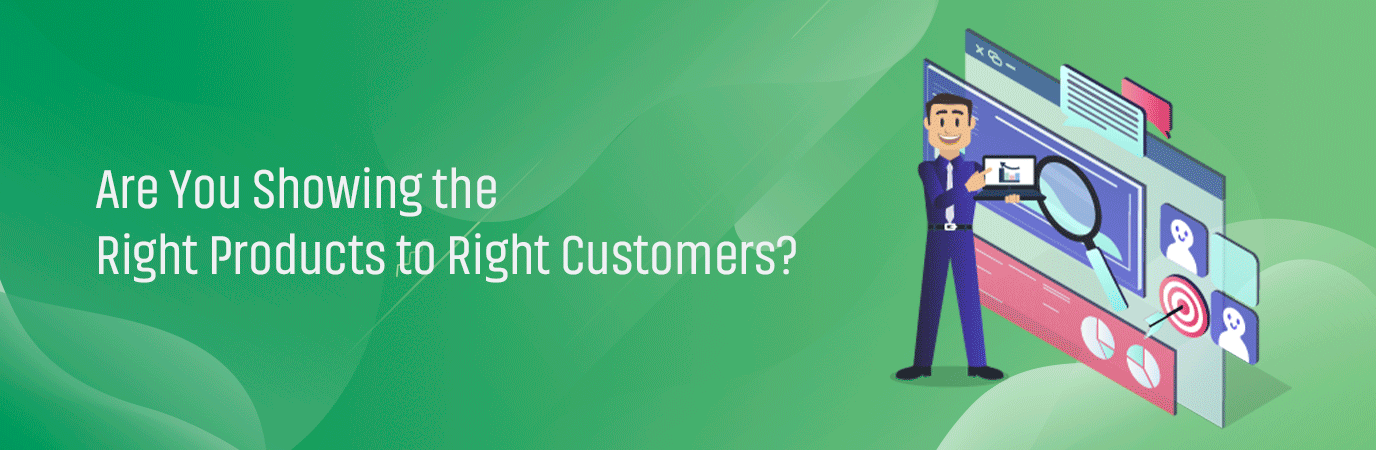
Add your comment