Unless you live under a rock, you already know Netflix is the most valued and sought after video streaming platform globally. The digital OTT landscape will forever owe to the streaming giant for cracking the secret to viewer engagement, viewing habits, patterns, and has inspired hundreds of companies to delve into the power of data. Netflix’s spectacular success will be incomplete without mentioning the sharper recommendation system it uses to recommend the right content to the right viewers – here, impeccable is an understatement.
As per research, Netflix recommender system algorithms produce $1 billion a year in value from customer retention. Another study suggests that people spend more time browsing the content library of Netflix than watching. That’s audience engagement on some other level. So, if you own an audio or video streaming service, you need to ensure that your viewers are served with the content they want to watch and not what you have in your library. Precisely, you need a recommendation system as good as Netflix to sustain in the race. Before we take you through the solution, you must understand how Netflix’s recommendation system works.
Five-Factor Recommendation Framework of Netflix
Viewer behavior or user behavior is defined as the interaction between the system or application and user at a given point in time. From tapping on a thumbnail to watching the trailer on Netflix, all your navigation is being captured and analyzed. Needless to say, viewer behavior or user behavior is the most critical parameter taken into account by any recommendation system.
- What Information Does the User Seek aka Content-based Filtering
Eighty percent of movies watched on Netflix come from recommendations, and deep learning is behind enhancing Netflix’s recommendation quality. DL typically uses deep neural networks (DNN) to derive movies or artist representations from the content metadata such as artist details or user-generated tags. These factors are then either directly used in Content-based filtering systems.
So, the next time you see the movies under the category “Because You Watched”, “Similar Shows”, “More like XYZ”, know that the recommendations are content-based and the reflection of what you might like as per your taste, preferences, and viewing history.
- Similar Viewers aka Collaborative Filtering
As the name suggests, millions of people are watching what you are watching on Netflix. Netflix analyzes each user’s data and the viewing pattern to recommend shows to a user with similar interests or viewing patterns. You often see a set of movies and NOT ALL under a specific category like “Action” or “Romance” or more. The collection of movies you find in that segment is a bespoke view for you and showcases the content of the users who have similar interests as you.
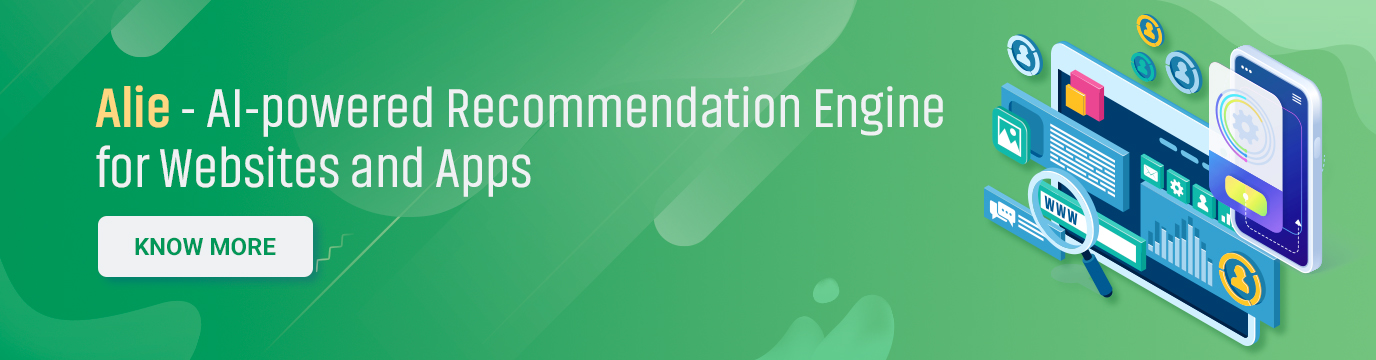
Contextual Information
Here is where Netflix excels. If you are logging in to Netflix on your mobile, there is a maximum probability that you will watch alone. So, don’t get surprised if you log in to your same account on your smart TV and get different recommendations. Because 43% of users who watch Netflix on smart TVs watch with Family and the recommendation varies.
Netflix’s time-based recommendations have proven to be a real content discovery tool. On weekends, viewers binge-watch. So, Netflix will showcase content that is binge-able on the weekend itself. Now the question arises, how does Netflix decide that? Well, the primary two parameters considered are the series duration and the no. of episodes.
|“Emily in Paris” is one of the most binge-watched series on Netflix on weekends.
You will always find Halloween, Thanksgiving, or Christmas themed movies and shows at the specific time of the year right on the top when you sign in with Netflix. Here, the recommendation works by the festivities around, and viewers end up watching shows they would never have searched for in the first place.
An image is worth a thousand words. If anybody has put this rightly to business, it’s Netflix. You will never find the same old posters of movies or series as thumbnails on Netflix. Netflix keeps them changing. For every new title, multiple images are assigned randomly to different subscribers from different communities. Netflix then picks the image that has the highest probability of getting clicked.
Also, a lot of contextual factors play a role in the artwork-trick. Netflix picks images with sequences like a dinner on the beach or an exciting car chase or a popular actor who is recently in the news, and the viewer has watched movies of his before. These factors combined hold the user’s attention and compel them to click to learn more about the title.
How to Recommend Content like Netflix?
As you understand how Netflix’s recommender system works in favor of it, you now realize its importance for any digital service. A recommendation engine utilizes Artificial Intelligence and machine learning to analyze data and recommend the right content to the right user in real-time. Here’s how Muvi’s ambitious AI-based recommendation engine Alie works in making impeccable recommendations to users.
Check out the features of Alie and how businesses are benefiting from it.
Unlock the power of recommendation using AI-based recommender system Alie. Take a Free Trial now and improve user engagement and click-through rate dramatically.
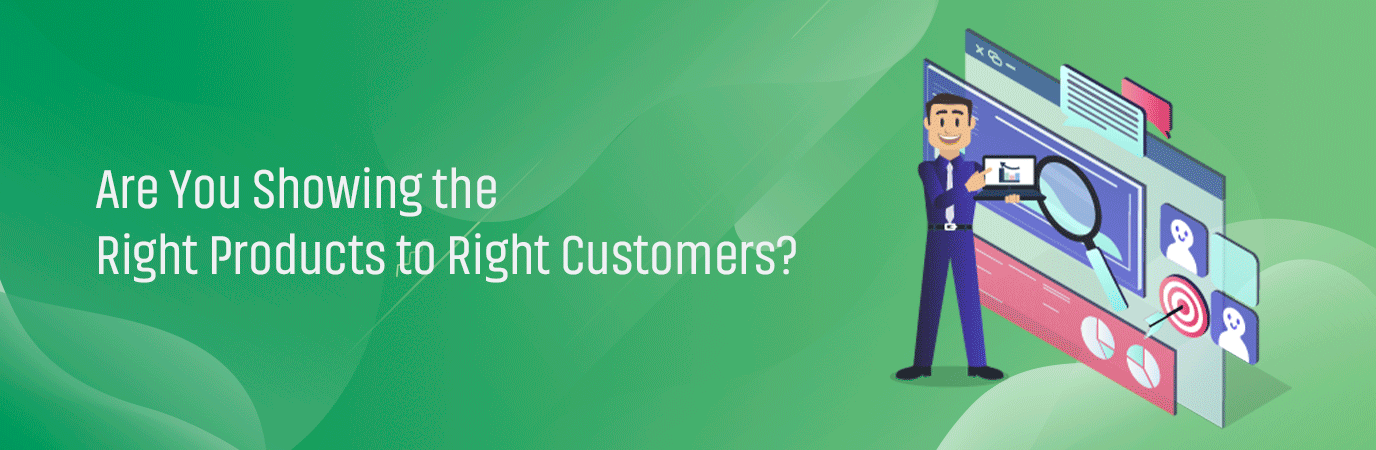
Add your comment