There are many people who start a business, but only those are called successful entrepreneurs who are able to expand their businesses. In the digital world, everyone knows that growing their business also means scaling your recommendation engine. There has been a lot of debate on how to scale a recommendation engine. Scalability is a significant problem with recommendation engines because calculations normally grow linearly with the number of users and items. Even the most efficient recommendation engine might fail to deliver relevant results when the volume of the dataset is high.
So, here are some of the few steps that one can take to scale a recommendation engine –
Switch to Item-Based Collaborative Filtering
One of the significant issues of collaborative filtering algorithms is that it faces the issue of scalability. With time as the businesses evolve, users in the websites increases. When the recommendations are made based on a user study, it requires more and more space. However, the items on the same website might be comparatively less than that of the users. So, making the switch from user-based to item-based collaborative filtering repositions the problem of accurate and timely recommendations to the item space. It helps explore the relationship between certain items instead of the relationship between users.
Also read : The difference between Collaborative and Content Based Recommendation Engines
Finding The Best Similarity Computation Technique
The concept of similarity is a crucial element of the collaborative filtering framework. In user-based collaborative filtering techniques, the user similarity index consists of a metric that calculates the distance between a pair of user preferences. Similarly, the item similarity index measures the similarities between a team or group of items in the item-based framework. The Three most commonly studied techniques are the Pearson, Cosine, and MSD (Mean Square Difference) methods. However, if you perform all these computation techniques, you will see the outputs are different for all three of them. So, it is better that you decide which kind of testing you want to use based on your set of data. Trying and making errors is the best way to find the best computation technique.
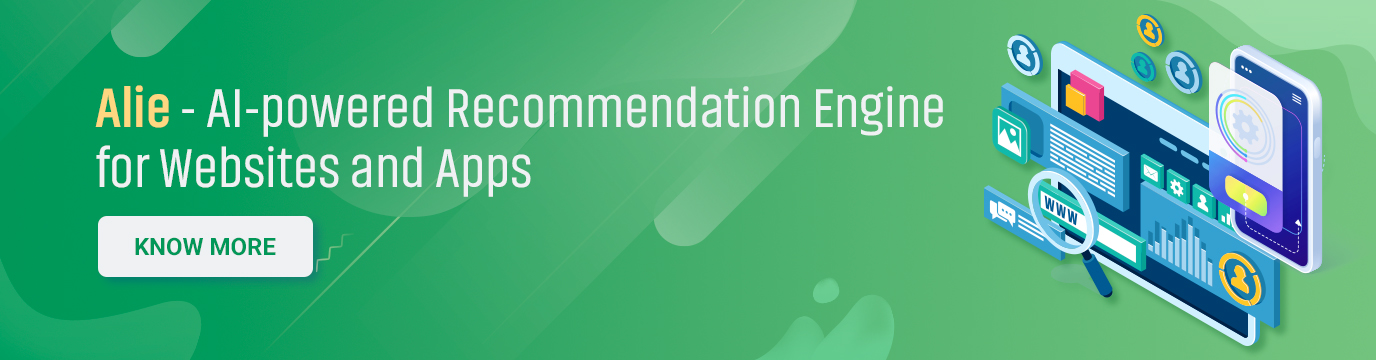
Amplifying Your Recommendation Engine Using Model Size
The model size is simply a neighborhood of the most similar items for a particular active item that has been rated by an active user. In an item-based collaborative filtering framework, the concept of model size concentrates on the idea that sufficient information about a user’s preference resides within a subset of similar items to the items they have already rated highly. Naturally, it makes sense that unviewed items that bear high similarities to the highest-rated viewed items contribute the most towards the final recommendations list in an Recommendation Engine.
With many items, compiling recommendations based on the complete data set in real-time becomes too computationally meticulous. Working on a ‘neighborhood’ of options reduces the time it takes to make a prediction and decreases the volume of information the model has to rank for the user. The critical trade-off, in this case, becomes the level of knowledge that ensures recommendations are both relevant timely.
Buy a Recommendation Engine that Supports Scalability
If your business needs the scaling of a recommendation engine once, then chances are you are going to need it again; and bravo, you are on your way to success. However, there are not many Recommendation Engine vendors who promise the option of scalability. So, look carefully before making your decision.
Wrapping Up
Scaling up a recommendation engine can become a little too hectic for many people. Although, one can achieve wonderful results with the proper steps. One should also try the recommendation engine platform – Alie, which offers the option of scaling up, and one can even choose which kind of collaborating filtering they want. The best part of Alie is that its user can see real-time recommendations on their platform, like the picture below. Try the 14-day free trial to know more about Alie and see for yourself its diverse features.

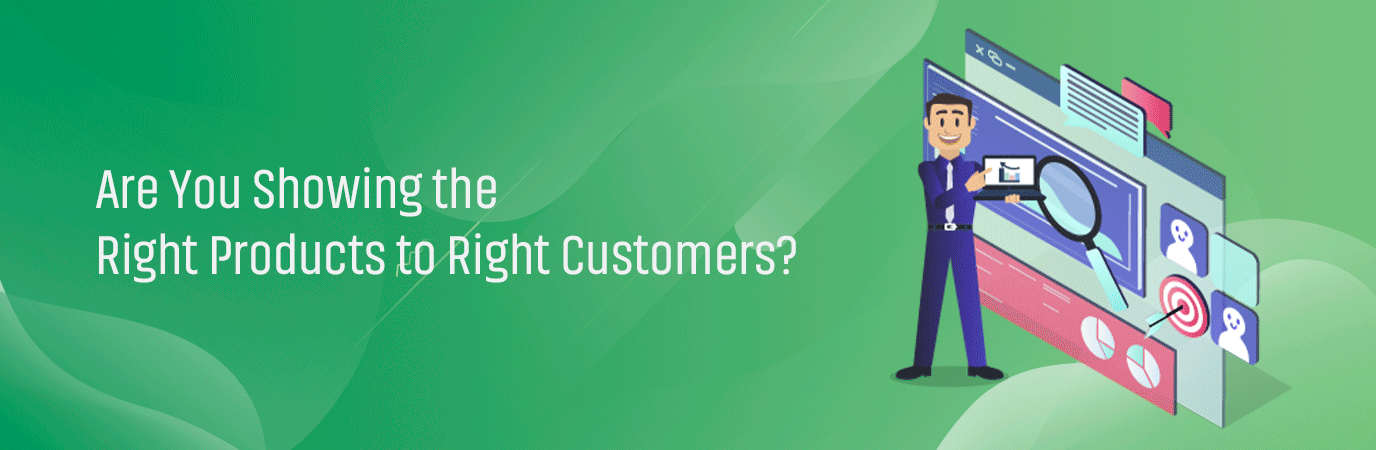
Add your comment