Since the dawn of internet, if there is one thing that is increasing exponentially besides bandwidth and speed, it’s information in the form of data. In the age of such information overload, if a person tried to search for something on the internet, presumably without using any sort of algorithms, then it might take him days to search and sort information of something as simple as specifications of a mobile phone. However, the internet as we know it is capable of delivering information in a matter of microseconds, owing to several tools and algorithms that have been developed over the years to make our lives easier. One such development is a recommendation engine.
The search for recommendation engines began back in the late 1970s at Duke University but it was first developed in 1992 at the Xerox Palo Alto Research Centre. It was developed to rate messages good or bad in the e-mailing system so that the messages can be segregated. Recommendation engines were further developed to help people select the most suitable product for them from the plethora of options available to them. The idea was developed from the fact that people often relied upon the opinions and reviews of their peers before trying something new, ex – buying a smartphone, laptop, before going for a movie, before going to a new restaurant and even before visiting a doctor. In today’s day and age, we are surrounded by numerous recommender systems starting from autocorrect features on your smartphone to movie suggestions on your favorite OTT platform.
What is a Recommendation Engine?
A recommendation engine is thus a tool that analyzes the behavior of users and takes into consideration various attributes, and then recommends the most appropriate suggestion a user may like to buy, watch or read(depending on the industry).
Some day-to-day life examples of recommendation engines are –
- The list of ‘Most buy Products’ on any eCommerce website like Amazon.
- The suggestion of ‘Most Popular Movies’ on an OTT platform like Netflix.
- The Auto-correction Application on your mobile phone.
Just like any other technology, even the recommendation engine had its own journey of shortcomings which were tackled through upgradations. In particular, these upgradations can be classified into three categories:
Three Generations of a Recommendation Engine
First Tier
The first-tier recommendation engine used all kinds of filtering techniques such as collaborative, content-based, and hybrid filtering so that the results obtained would be perfect. However, even after using multiple filters, the first-tier recommendation engines proved only fruitful for the e-mail system. Some of the advantages and disadvantages of a first-tier recommendation engine are –
Second Tier
The second Tier recommendation engine heavily relied on the tags to recommend products. Tags here mean the special label given either to the user or to the product. As a collective, the second-tier proved to be useful in giving high-quality tag recommendations but failed in using the correct information, and in the long run, it also resulted in information loss. Advantages and disadvantages of Second Tier recommendation engine are –
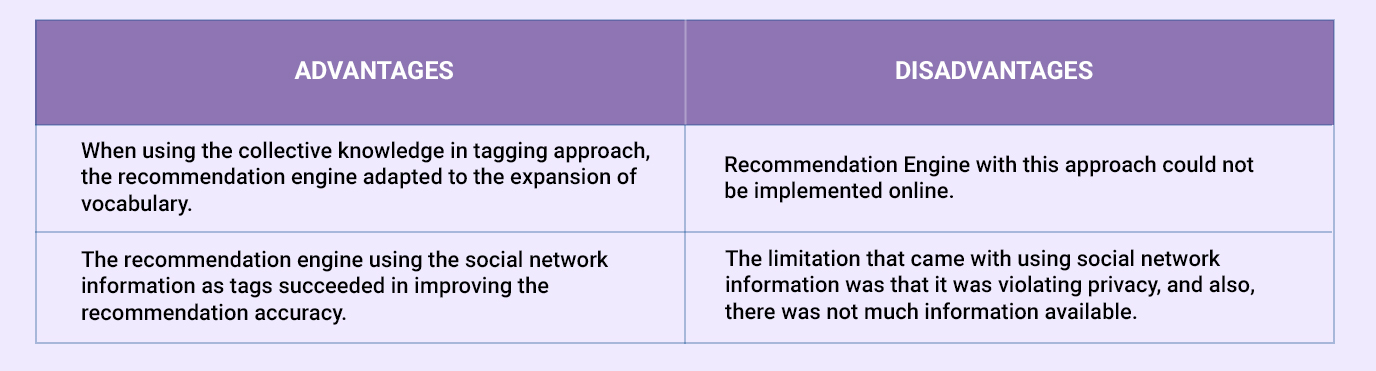
Third Tier
The third-tier recommendation engine is the latest version and the smartest one till now. It came into existence mainly after the increased usage of mobile phones. It uses Artificial Intelligence and the Internet of Things to recommend products to users. Here’s a list of advantages and disadvantages of a third-tier recommendation engine system –
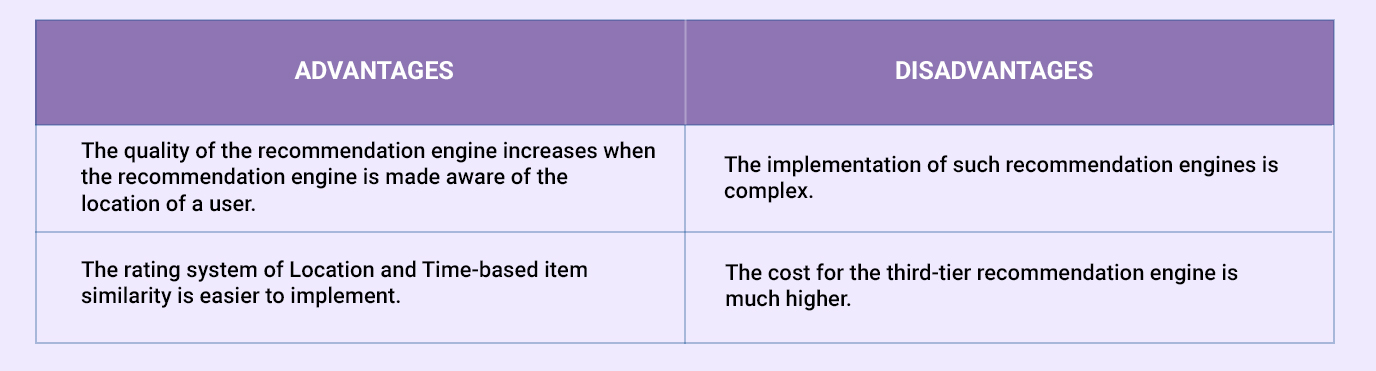
Wrapping Up
Having learned the different generations of recommendation engines and the advantages and disadvantages that come along with them, one thing can be clearly said – recommendation engines are complex. However, what if you don’t have to build a recommendation engine in order to integrate it with your website and leverage it? All you have to do is explore different recommendation engines that are available in the market and choose the best one for your website. Alie – an AI-based recommendation engine, for instance, is a third-tier recommendation engine that uses Artificial Intelligence and IoT to recommend products. However, it also gives the liberty to its customer to pick from the first and second tier of recommendation engines. The best part about Alie is that you can also see which product is being recommended to which user.
If you found this information useful and want a world-class next gen recommendation engine on your site as well, We recommend you to try out our 14-day free trial of Alie and enable your users with the power of AI to explore your website better.
Add your comment